The Evolution of AI Agents: From Simple Tasks to Advanced Problem-Solving
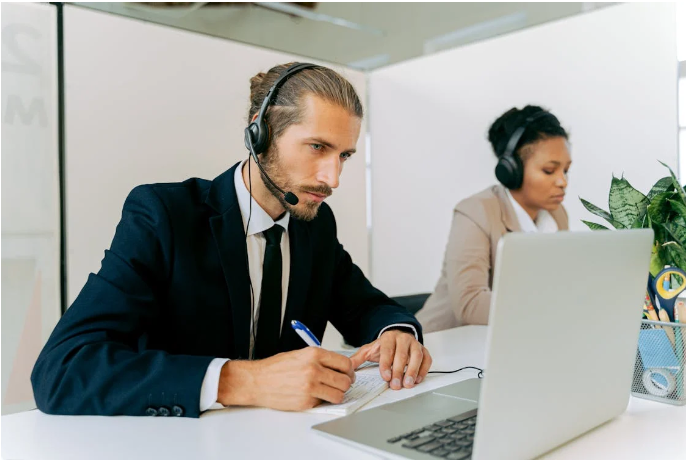
The Evolution of AI Agents: From Simple Tasks to Advanced Problem-Solving
In the rapidly advancing field of artificial intelligence, AI agents have emerged as one of the most transformative technologies of our time. These intelligent software entities have evolved from performing basic, predefined tasks to tackling complex problems with human-like reasoning capabilities. This article explores the fascinating journey of AI agents, tracing their development from humble beginnings to their current status as sophisticated problem-solvers.
The Genesis of AI Agents
The concept of AI agents dates back to the early days of artificial intelligence research in the 1950s and 1960s. Initially, these agents were simple programs designed to perform specific, narrow tasks. They operated on a set of predefined rules and lacked the ability to learn or adapt to new situations.
One of the earliest examples of an AI agent was the Logic Theorist, developed by Allen Newell, Herbert A. Simon, and Cliff Shaw in 1955. This program was designed to prove mathematical theorems and is often considered the first artificial intelligence program. While groundbreaking for its time, the Logic Theorist was limited in scope and could only operate within a narrow domain of mathematical logic.
The Rise of Expert Systems
As AI research progressed through the 1970s and 1980s, a new type of AI agent emerged: expert systems. These were computer programs designed to emulate the decision-making ability of human experts in specific fields. Expert systems relied on a knowledge base of facts and rules, coupled with an inference engine to apply this knowledge to solve problems.
MYCIN, developed at Stanford University in the early 1970s, was one of the most famous expert systems. It was designed to diagnose blood infections and recommend appropriate treatments. While MYCIN demonstrated impressive capabilities within its domain, it still lacked the ability to learn from experience or adapt to new situations outside its programmed knowledge base.
The Machine Learning Revolution
The 1990s and early 2000s saw a paradigm shift in AI agent development with the rise of machine learning. Instead of relying solely on predefined rules, AI agents could now learn from data and improve their performance over time. This marked a significant leap forward in the evolution of AI agents, as they could now tackle more complex and diverse tasks.
One of the most notable developments during this period was the creation of IBM’s Deep Blue, a chess-playing computer that famously defeated world champion Garry Kasparov in 1997. While Deep Blue was still primarily rule-based, it incorporated machine learning techniques to evaluate chess positions and select optimal moves.
The Deep Learning Breakthrough
The advent of deep learning in the 2010s revolutionized AI agent capabilities. Deep learning, a subset of machine learning based on artificial neural networks, allowed AI agents to process and learn from vast amounts of unstructured data. This breakthrough enabled AI agents to excel in tasks that were previously thought to be the exclusive domain of human intelligence, such as image and speech recognition, natural language processing, and complex decision-making.
A landmark moment in this era was the development of AlphaGo by DeepMind, which defeated world champion Go player Lee Sedol in 2016. Unlike chess, Go was considered too complex for traditional AI approaches due to its vast number of possible moves. AlphaGo’s success demonstrated the power of deep learning and reinforcement learning in tackling highly complex problems.
The Era of Multi-Modal AI Agents
As we move into the 2020s, AI agents are becoming increasingly sophisticated and versatile. Modern AI agents can integrate multiple types of data and perform a wide range of tasks, from natural language understanding and generation to visual perception and reasoning.
GPT-3 (Generative Pre-trained Transformer 3), developed by OpenAI, exemplifies this new generation of AI agents. It can engage in human-like text conversations, answer questions, write essays, and even generate code. The ability of GPT-3 and similar models to understand context and generate coherent, relevant responses marks a significant step towards more general artificial intelligence.
AI Agents in Real-World Applications
The evolution of AI agents has led to their widespread adoption across various industries and domains. In healthcare, AI agents are assisting in diagnosis, drug discovery, and personalized treatment plans. In finance, they’re being used for fraud detection, algorithmic trading, and risk assessment. In customer service, AI-powered chatbots and virtual assistants are handling increasingly complex queries and tasks.
One particularly exciting application is in autonomous vehicles. AI agents in self-driving cars must integrate data from multiple sensors, make split-second decisions, and navigate complex environments. Companies like Tesla, Waymo, and Uber are at the forefront of this technology, pushing the boundaries of what AI agents can achieve in real-time, safety-critical applications.
The Future of AI Agents: Towards Artificial General Intelligence
As impressive as current AI agents are, they still fall short of human-level intelligence in many aspects. The holy grail of AI research is Artificial General Intelligence (AGI) – AI systems that can match or exceed human intelligence across a wide range of cognitive tasks.
While AGI remains a distant goal, researchers are making steady progress. Projects like OpenAI’s GPT series and DeepMind’s AlphaFold are pushing the boundaries of what AI agents can achieve in language understanding and protein folding, respectively. These advancements suggest that future AI agents may be capable of even more complex problem-solving and reasoning.
Ethical Considerations and Challenges
As AI agents become more advanced and integrated into our daily lives, important ethical and societal questions arise. Issues of privacy, bias, accountability, and the potential impact on employment are at the forefront of discussions among researchers, policymakers, and the public.
Ensuring that AI agents are developed and deployed responsibly is crucial. This includes addressing biases in training data, ensuring transparency in decision-making processes, and establishing clear guidelines for the use of AI in sensitive areas such as healthcare and criminal justice.
Conclusion
The evolution of AI agents from simple, rule-based programs to sophisticated problem-solvers has been nothing short of remarkable. Today’s AI agents can perform tasks that were once thought to be the exclusive domain of human intelligence, and their capabilities continue to expand at an astonishing pace.
As we look to the future, the potential applications of AI agents seem limitless. From revolutionizing scientific research to transforming industries and enhancing our daily lives, AI agents are poised to play an increasingly central role in shaping our world.
However, as we continue to push the boundaries of what AI agents can do, it’s crucial that we remain mindful of the ethical implications and potential risks. By fostering responsible development and deployment of AI technologies, we can harness the full potential of AI agents while ensuring they benefit society as a whole.
The journey of AI agents from simple tasks to advanced problem-solving is far from over. As research continues and new breakthroughs emerge, we can expect AI agents to become even more capable, versatile, and integrated into our lives, opening up new possibilities and challenges for humanity to explore.